News
Artificial Intelligence Enables High Recognition Rate of Tropical Cyclone Precursors - Dangers of Cyclones Could be Spotted Early through Deep Learning of Cloud Images Updated in February 2019
Recently, there has been a rapid increase in research to apply artificial intelligence (AI) to meteorology. One way is to identify image patterns by deep learning. “Until about a year ago, I’ve been trying to have AI do things in place of humans, but now we’re beginning to explore the possibility for AI to do things that humans cannot,” said Daisuke Matsuoka of Japan Agency for Marine-Earth Science and Technology (JAMSTEC), an expert on information processing in this field. Matsuoka’s team recently published a paper on AI spotting precursors or very early stages of tropical cyclones.
So far, tools used in the study of weather, like global warming and typhoons, are mainly based on physics, particularly dynamics. When a force is applied to an object, how would the object change its speed and in which direction? Such changes are analyzed and organized in the form of equations. Because the center of the typhoon is low in pressure, air from the outside would gush inwards. Then, what would happen to the atmosphere? There are many types of mathematical formulas used to predict typhoons, but they are very complex. However, they are based on a causal relationship; when there is a specific cause, a certain phenomenon would occur as a result. This is exactly the concept of dynamics.
Now, pattern-recognition through AI is being introduced. Deep learning is not based on causal relationships.
Small children love vehicles. In time, they will be able to distinguish between trains and automobiles as they get used to seeing them. An adult does not have to teach the difference between the two: “A train runs on two rails and gets electricity from the overhead wire while a car can drive on roads without rails and has four black tires.” Lengthy explanations are unnecessary. Such a process to naturally digest differences is called learning. Even if you do not know what caused the child to distinguish between a train and a car, the child will eventually be able to do so. Deep learning is to have the computer imitate such functions of the human brain. It is completely different from “physics” where one is driven to pursue the ultimate cause.
Traditional meteorology and deep learning do not exactly go hand in hand. But in real-life typhoon forecasts, they already coexist. Offshore typhoons which cannot be spotted precisely by observation equipment are determined by the human eye based on cloud shape images captured by meteorological satellites.
This so-called “Dvorak method” (developed in 1974 by the American meteorologist Vernon Dvorak) is a pattern-recognition technique created through human learning. “If we let AI do such recognition, we might be able to find precursors or small signs of tropical cyclones,” thought Matsuoka’s team.
For machine learning, Matsuoka and his colleagues used computer-simulated global weather condition data worth 20 years. This data includes reproduced tropical cyclone clouds. From this sea of data, the team prepared 50,000 images of cyclone precursors and growing cyclones. There were also 1 million images of clouds which did not turn into cyclones. From the 1 million non-cyclone images, 10 sets of 50,000 images were each combined with the very first batch of 50,000 cyclone-cloud images. In other words, 10 sets of images, each including 50,000 sheets of tropical cyclone images and 50,000 non-cyclone images were put together. The same cyclone-images (50,000 sheets) were used in all 10 sets.
Using the 10 sets of data, the team created 10 types of “tropical cyclone classifiers” by using AI. Each classifier read 100,000 cloud images to learn whether an image constituted a tropical cyclone or not. By viewing completely new cloud images later, the classifier must determine whether they resemble a tropical cyclone or not. Because each classifier went through learning under different data, their ability to recognize tropical cyclones varied. The final decision was made through comprehensive evaluation as if 10 people had made judgements after looking at a specific cloud image.
Consequently, when 10 classifiers recognized a tropical cyclone or its early stage, 8 real cyclones out of 9 were detected correctly. That is a recognition rate of about 90 percent. An early sign which later developed into a tropical cyclone in 3.5 days was distinguished correctly. The rate for incorrect recognition was about 10 percent.
From observation, the team found out that better recognition is possible in oceanic areas where cyclones are more frequent and long-lasting. The Northwest Pacific during summer to autumn brought better results.
According to Matsuoka, AI’s cyclone recognition rate is higher than human judgement. However, the study used simulated data which include supportive information besides cloud images. From this aspect, the AI classifier had some advantages compared to recognition through real satellite images only. This study showed that AI could be used to detect tropical cyclones. Matsuoka and his team are also considering methods on how to achieve precise recognition based on real observation data.
https://progearthplanetsci.springeropen.com/articles/10.1186/s40645-018-0245-y
https://www.jamstec.go.jp/e/about/press_release/20181219/
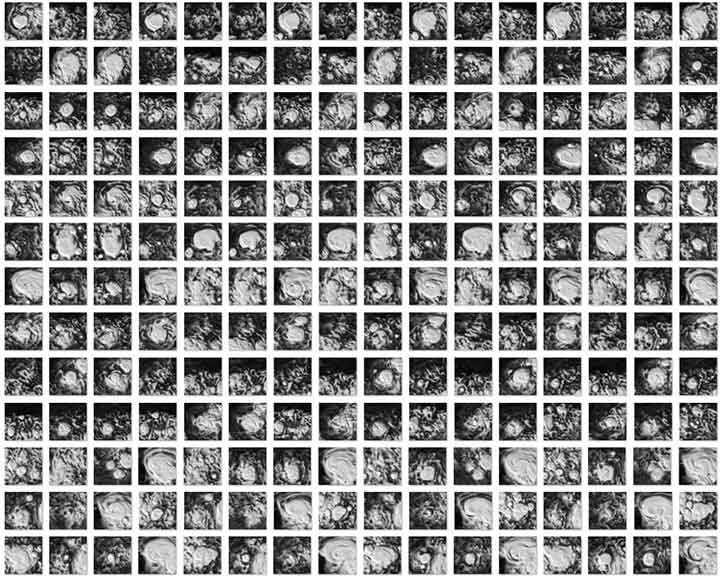